ARTICLE: Generative AI as a bottomless pit for the culture community?
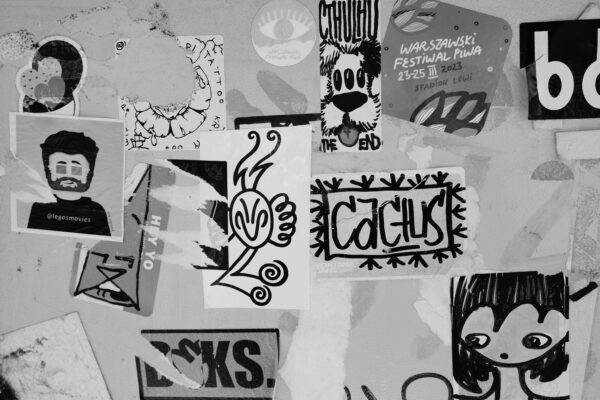
Anielek Niemyjski
Kacper Solecki
The Warsaw Observatory of Culture (WOK) has been studying the transformation of cultural practices in the age of the digital revolution. The recently published report on the readership of young Varsovians is just one example of its commitment to the issue. Our interest in the processes we participate in, in this case, digitisation led us to explore narratives about cultural institutions during infrastructural change, or at least one particular part of it, namely the increased interest in artificial intelligence. In the season of the “artificial intelligence boom”, why not draw on the knowledge gained from recent conversations about the established (and emerging) models of cultural work – ecology and overproduction?
When the topic of “AI in culture” comes up, we tend to hear about artistic objects created with the help of generative AI-based tools. We also hear about the challenges of copyright reform when cultural data is used to train neural networks. From this perspective, cultural institutions tend to fade into the background. Meanwhile, the people working in the institutions we focus on in this article face challenges similar to those faced by professionals in other sectors, especially regarding the legal and ethical framework for collecting, developing and using new solutions. Talking about working conditions can enrich the conditions that focus on artists and artistic production.
A noticeable boom in the training of cultural personnel, new courses are emerging that promise institutions and staff “acquire real 21st century competencies”’ and “learn about real trends and future technologies”. We were intrigued by the circulating narratives of innovation that saturate ads and descriptions of educational offerings. We believe this is an excellent lens through which to analyse how the topic of digitalisation manifests itself both within institutions and in industry discussions. By asking what emerges most frequently in “discussions about AI in culture”, we seek to identify the threads that are not being addressed, namely the indifference of the debate to recent conversations about the current work model and productivity in the cultural sector.
The innovation narrative
Training courses offer the opportunity to acquire skills and experience in working with tools “of infinite possibilities”, i.e. the now widely available, ever newer versions of software such as ChatGPT or Adobe Firefly. We have probably all encountered similar themes: generative AI being presented as an intangible (i.e., often ‘free’) assistant that will relieve us of the most tedious tasks, or as a tool that will simplify specific procedures. The solution is meant to address pressing needs of institutions, facilitate digitisation and provide digital access to collections for groups with diverse needs. Perhaps most interesting from the perspective of an institution’s day-to-day operations is the widespread use of publicly available, partly free language models such as Open AI’s ChatGPT. According to the training narrative, we learned that a simple-to-use chatbot, after entering specific prompts (commands), can help us write a grant proposal, create a social media event communication, or write a script for a library lesson. According to the innovation narrative, generative AI tools resemble a bottomless pit offering infinite ways to tackle tedious tasks so that we can devote more time to other activities.
Discussions around these tools hover between the promise of streamlining everyday work and concerns that their implementation could lead to job losses. However, in reality, the only challenges and constraints being addressed in the innovation narrative are the legal and ethical conditions for data processing (to which the AI ACT and the Artificial Intelligence Liability Directive at EU level are intended to respond). Discussions of alternatives to the current “overproductive” model of organisation and management in cultural institutions, intensified by the COVID-19 pandemic, seem to escape such a framework of the debate.
Nadprodukcja
In today’s discourse on artificial intelligence, we often hear that it is an ineffectual tool that can help us adapt to the current rhythm of work, i.e. overproduction, characterised by “[the] penetration of market mechanisms into all areas of our lives, fields of creativity, the institutions in which we work”[1]. To paraphrase Weronika Parafianowicz, it is about constant movement, for example, the uninterrupted execution of successive projects, often without evaluation of their content, and the flexibility of employees constantly extending their competencies and being given responsibilities beyond their capacity. As we observed, the COVID-19 pandemic highlighted the weaknesses of such a working model. Therefore, there has been considerable interest in rethinking how we can “work in culture”.
The lack of discussion of overproduction in the debate about using AI-based solutions seems to have affected our perceptions and hopes for these tools. We tend to use them to maintain the current productivity model, with the assurance that “generative AI will take the strenuous tasks out of our hands” and that we can and will get on with the work we enjoy, such as creative work. Sounds like a dream, doesn’t it? However, it is worth considering whether these visions are even feasible. Will artificial intelligence free us from our daily work, or will the responsibilities of institutional employees continue to expand as everyone gains access to an artificial “personal assistant”? In this discussion, there are also rare voices that raise the current conditions of working with AI-based tools: the limited possibilities of using paid versions and the varying effects of working with free tools (for which we pay with access to our data). Overproduction is characterised not by the accumulation of staff skills, but by their dissolution. Having to expand their knowledge into newer areas prevents cultural workers and employees from deepening their competences.
(In)finite natural resources
When learning about the benefits of generative artificial intelligence in the workplace, its impact on the environment is often treated as a secondary concern. No wonder we are initially spellbound by AI’s benefits, such as process automation, photo and video creation and editing tools, and advanced data analysis. Also, we are only just finding our way around the ever-changing versions of ChatGPT and discovering its functions in our professional and personal lives.
Unfortunately, this ever-increasing interest in artificial intelligence’s possibilities is increasingly influenced by environmental consequences. In this case, intangibility seems to refer only to the realm of exciting technology, as research into its impact on the ecosystem exposes a dramatic problem.
In approximating data on generative AI’s environmental impact, we will mainly rely on research conducted on the OpenAI tool, due to its popularity and the availability of these studies and calculations. One of the current problems in estimating the actual impact of available types of generative AI on the environment is that they are owned by private companies, so the exact data and their specifications are not publicly available.
It is estimated that one ChatGPT query generates 4.32 grams of carbon dioxide.[2] Of course, before such a tool is ready to accept user commands and queries, it needs to be trained, i.e. fed with data. A 2021 study found that training a ChatGPT-3 model generated 502 tonnes of carbon dioxide,[3] equivalent to the average emissions of 112 internal combustion cars in a year.[4]
Unfortunately, carbon emissions and energy consumption in data centres are not the only costs of artificial intelligence to the environment and, above all, to humanity.
Another relatively recent impact of AI development is increased water consumption. In daily operation, AI servers in data centres generate significant heat and require cooling. It has been shown that approximately 5.4 million litres of water are used to train an AI model such as GPT-3, with an additional 500 ml of water used for every 10-50 queries.[5]
The next, higher, and more powerful chat model, GPT-4.0, was released in May 2024. This is crucial information as each successive version of the tool is more complex and has a successively greater environmental impact. We do not know what water consumption or atmospheric carbon emissions the new ChatGPT model is responsible for. Many researchers are calling for transparency about the environmental impact of new AI models and tools.
Without accurate data, we will not be able to control the impact of AI on the environment and thus anticipate the alarming use of natural resources essential for sustainable life on Earth.
Emotions
The noticeable growth of interest in artificial intelligence in the cultural environment, especially in the public sector, seems to lack concern for those employed in it. Given the constant onslaught of the technological revolution, we tend to forget about those experiencing technological exclusion. We should not allow a situation where working people are burdened with additional responsibilities, that are downplayed by the to do them through ChatGPT. The chat tool is helpful, but it often requires more than just scripting a prompt to get the desired result.
In the eyes of many employees, culture is a space that prioritises emotion, interpersonal relationships and creative interaction, which can create doubts about technology that seems more impersonal and distant from human values. However, such reservations, which combine excitement with apprehension, often co-exist, so it is crucial that institutional managers not only understand these concerns but also adequately support employees in the adaptation process so that AI can be implemented effectively and smoothly, benefiting both teams and the institution as a whole.
Summary
Among the many hot topics in culture, we decided to highlight the use of generative AI in the work of the public cultural sector. In the general debate on artificial intelligence, we wanted to include topics that may have been marginalised. We believe that the issues of overproduction and lack of transparency about the environmental impact should be explicitly highlighted. At the same time, we must not forget the emotions of employees, for whom the implementation of new technologies poses significant challenges. Support and open communication about changes in the workplace should become a priority, so that the process of implementing AI benefits not only the institutions but also the people who work in them.
Instead of a technophobic attitude that assumes absolute scepticism towards new processes, we propose the practice of recognising which needs and problems, especially those we often attribute to automation, but not only – at the level of the institution, our daily work, and the cultural field are to be answered by progressive digitalisation. What systems of interrelationships of expectations, recognitions, uncertainties and difficulties will we be able to map if we try to open up the discussion on innovation a little?
[1] Weronika Parafianowicz, “Nadprodukcja” [Overproduction]. Dialog. Miesięcznik poświęcony dramaturgii współczesnej, 22.08.2024, https://www.dialog-pismo.pl/w-numerach/nadprodukcja.
[2] Vinnie Wong, “Gen AI’s Environmental Ledger: A Closer Look at the Carbon Footprint of ChatGPT,” Piktochart, 3 May 2024, https://piktochart.com/blog/carbon-footprint-of-chatgpt/
[3] David Patterson et al.,” Carbon Emissions and Large Neural Network Training” (arXiv, 23 April 2021), [http://arxiv.org/abs/2104.10350]
[4]Renée Cho, “AI’s Growing Carbon Footprint”, Columbia Climate School, 9 czerwca 2023, https://news.climate.columbia.edu/2023/06/09/ais-growing-carbon-footprint/.
[5] Pengfei Li i in., „Making AI Less «Thirsty»: Uncovering and Addressing the Secret Water Footprint of AI Models” (arXiv, 29 październik 2023), [http://arxiv.org/abs/2304.0327]
Selected references:
Cho, Renée. “AI’s growing carbon footprint”, Accessed on 9.06.2023. https://news.climate.columbia.edu/2023/06/09/ais-growing-carbon-footprint/,
Crawford, Kate. Atlas of AI. Power, politics, and the planetary costs of artificial intelligence. New Heaven: Yale University Press, 2021.
Li, Pengfei, Jianyi Yang, Mohammad A. Islam i Shaolei Ren. “Making AI Less “Thirsty”: Uncovering and Addressing the Secret Water Footprint of AI Models”. Arxiv.org, 04 2023.
Lis, Bartek, Jakub Walczyk. “Wszechpraca i nadprodukcja w kulturze. Okołopandemiczne refleksje na marginesie badań pracowników i pracownic poznańskiego pola kultury” [Omniwork and overproduction in culture. Circumstantial reflections on the margins of a study culture workers in Poznań]. Zarządzanie w kulturze 22. No. 2(2021): 141-157.DOI:10.4467/20843976ZK.21.010.13764.
Parafianowicz, Weronika, “Nadprodukcja” [Overproduction]. Dialog. Miesięcznik poświęcony dramaturgii współczesnej. Accessed on 22.08.2024. https://www.dialog-pismo.pl/w-numerach/nadprodukcja.
Patterson, David, Joseph Gonzalez, Quoc Lee, Chen Liang, Lluis-Miquel Munguia, Daniel Rothchild, David So, Maud Texier and Jeff Dean. “Carbon Emissions and Large Neural Network Training”. Arxiv.org, 04.2021. https://arxiv.org/ftp/arxiv/papers/2104/2104.10350.p.
Przegalińska, Aleksandra, Leon Ciechanowski. Wykorzystywanie algorytmów sztucznej inteligencji w instytucjach kultury. [The Use of AI Algorithms in Culture Institutions] A study developed at the request of the Ministry of Culture and Cultural Heritage, Warsaw: Ministerstwo Kultury i Dziedzictwa Narodowego, 2020.
Ślęzak, Ida. “Ekologia w instytucjach kultury”, grotowski.net. Accessed on 22.08.2024. https://grotowski.net/performer/performer-19/ekologia-w-instytucjach-kultury,
Wong, Vinnie. “Gen AI’s Environmental Ledger: A Closer Look at the Carbon Footprint of ChatGPT”. Grafiati.com, 3.05.2024. https://piktochart.com/blog/carbon-footprint-of-chatgpt/